
Tim is currently studying for a PhD with Professor Richard Green. He is involved with two projects: Precision Farming for Aquaculture (Cawthron/Chris Cornelisen) and Transforming Scallop fishing: Non-destructive surveying and harvesting for economic acceleration and kaitiakitanga (NIWA). As well as his PhD, Autonomous identification and sizing of scallops in situ (NIWA).
“I enjoy developing robotics and AI with real-world impacts and enjoy the challenge of implementing advanced computer vision in the wild.
We’ve encountered plenty of life in the ocean, including schools of fish, sting rays, octopuses, and Hector’s dolphins.
It’s been a privilege to work in stunning locations around New Zealand alongside expert marine biologists.
Implementing computer vision underwater presents many challenges including keeping water out, having to debug on a rocking boat, and dealing with complex image distortions.” Tim
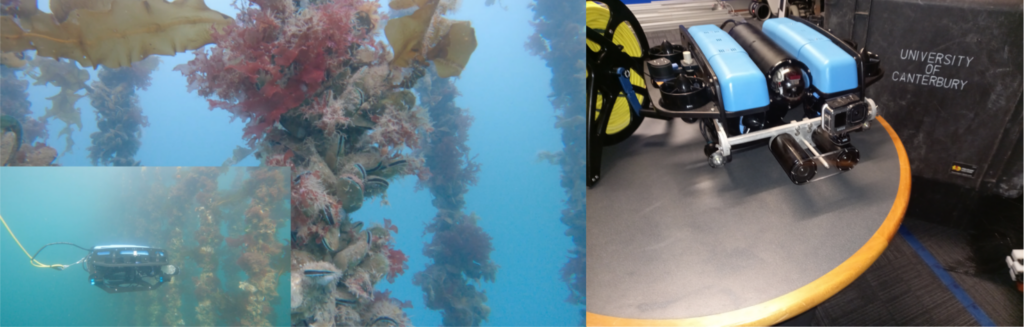
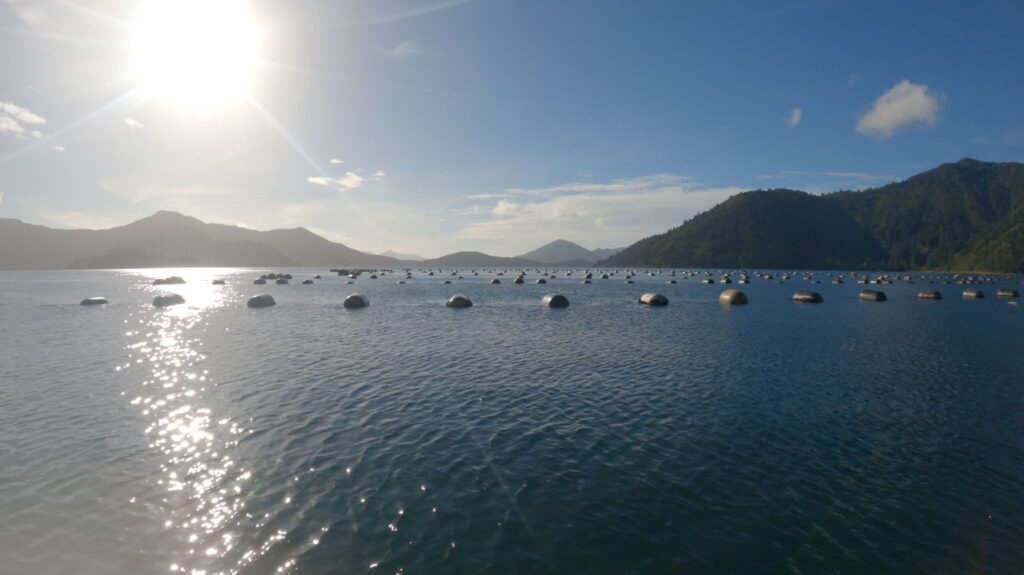
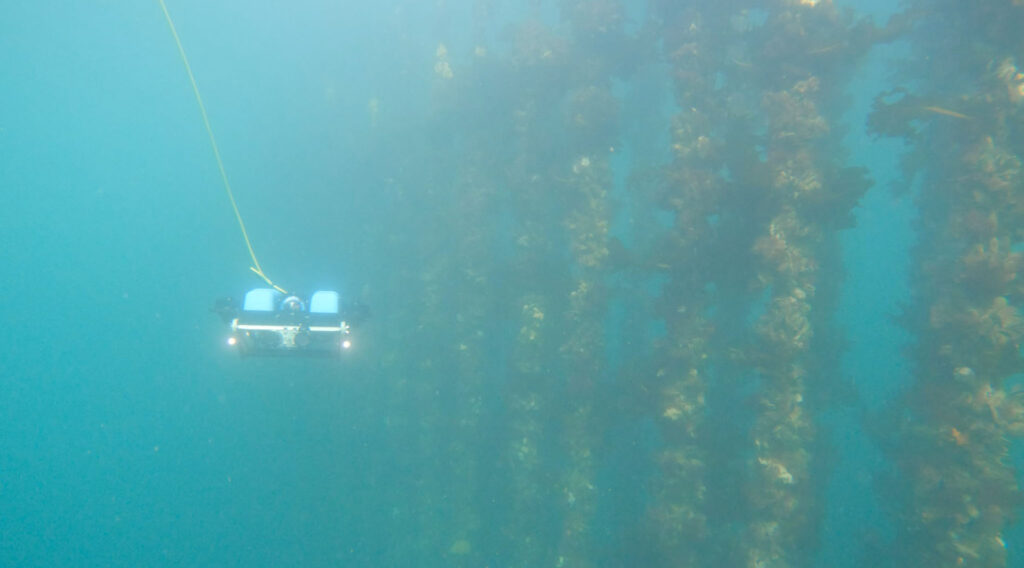
Scallop harvesting and stock monitoring with robots
We are facing a crisis in seafood sustainability! Many species of sea-creature are relied upon for food and livelihood, one of which is New Zealand’s endemic cryptic scallop.
● The southern scallop fishery, including Golden Bay, Tasman bay, and the Marlborough sounds was closed due to stock collapse in 2017, halting commercial and recreational fishing of hundreds of tonnes of scallops, and rahui or restricted access imposed on customary fishing by local Maori.
● Many factors contributed to this stock collapse, including overfishing and damaging fishing practices, sediment and nutrient runoff from land use, and natural events such as once-in-a-hundred-year floods turning productive seabed into sediment muck meters deep, suffocating and starving seabed communities.
● Scallop stocks are currently being monitored by seabed transect surveys, which are labourious and costly when done by human divers.
● Commercial harvesting is done by dredging, which involves indiscriminately raking up scallops from shallow seabed where they reside, damaging the habitat and negatively impacting future harvests. A proportion of scallops in the path of the dredge suffer fatal injury, even though they are not collected.
● For commercial scallop fishing to be sustainable we need better harvesting and monitoring methods. Using underwater robots, we can scan large areas of seabed consistently. The image data is processed using 3D reconstruction, allowing scientists to analyse entire habitats with a single 3D model or mosaic image as opposed to frame-by-frame in a video.
● To improve the efficiency of stock monitoring we are developing neural networks to automatically detect scallops in images. The scallop detector is trained using large datasets of images with labelled scallop pixels, aiming to capture as much variation in perspective, image quality, and environment as possible.
● We reduce the required size of the training dataset by using semi-supervised machine learning techniques such as image augmentation- which approximates different camera perspectives and image qualities, and label propagation, which uses camera position estimations to propate labelled pixels to nearby unlabelled views.
● The detected scallop pixels can be processed to gain the exact position and sizes of scallops without disturbing them.
● Eventually, our robot will detect harvestable scallops in real-time and selectively pick them up, eliminating by-catch and minimising habitat damage.
● The result would be more thorough and effective environmental monitoring and sustainable scallop harvesting, enhancing kaitiakitanga, our stewardship of earth.
